时间:2022年4月29日 星期五 下午14:00—18:00
地点:腾讯会议ID: 917 437 335 重庆大学虎溪校区理科楼LE201
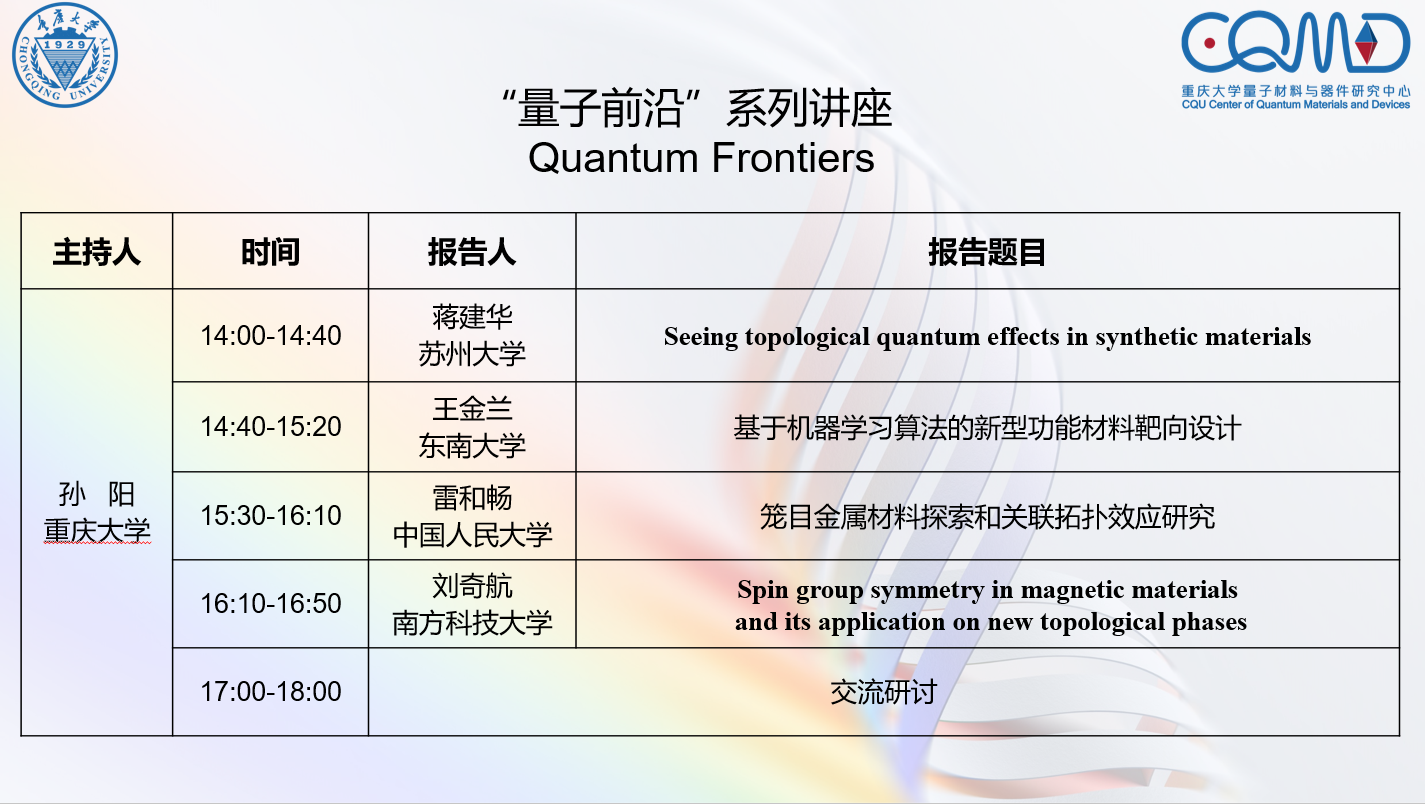
Seeing topological quantum effects in synthetic materials
主讲人:蒋建华教授
报告摘要:
Recent years have witnessed the vigorous researches on novel topological quantum physics in synthetic lattice systems, such as in cold atom systems and in metamaterials. I will show that metamaterials as synthetic crystalline materials can realize unprecedented topological quantum phenomena at nonequilibrium. These include the bulk-disclination correspondence which could serve as a new probe of topological crystalline materials and extremely local gauge flux manipulation which could provide a pathway toward fundamentally new materials and phenomena. We demonstrate all these effects with complimentary theory and experiments and pointing out its relation with physics in solid state materials.
主讲人简介:
蒋建华,苏州大学教授。2000-2010年在中国科学技术大学学习,获得学士和博士学位。2010-2012年在以色列Weizmann研究所凝聚态物理系跟随沃尔夫奖得主Yoseph Imry教授从事博士后研究。2013-2015年在加拿大多伦多大学物理系跟随Sajeev John教授从事博士后研究。2015年起任苏州大学教授。2018年获得江苏特聘教授。入选2019年批次教育部国家级青年人才项目。2021年获得国家杰出青年基金资助。主要从事拓扑光子学和声子学、量子和非平衡统计物理研究。创新发展了利用室温光子和声子系统实现新颖拓扑物理现象的研究。特别在拓扑体-缺陷对应关系、高阶拓扑物质态、非阿贝尔拓扑态等领域做出了开创性的研究。为拓扑光子学和拓扑声子学的发展做出了重要的贡献。在国际和全国会议上作大会和邀请报告共二十几次。发表同行评审学术论文90余篇,其中通讯作者论文含Nature、Nature Physics、Nature Materials、Nature Review Physics、Nature Commun.、Phys. Rev. Lett.、Phys. Rev. X、Science Bulletin等。
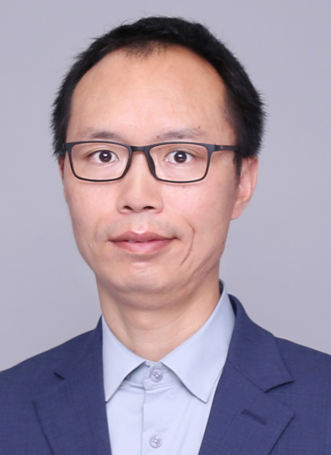
基于机器学习算法的新型功能材料靶向设计
主讲人:王金兰教授
报告摘要:
功能材料的研发是工业创新的基石,如何按需高效构筑材料一直是科学研究的热点与难点,尤其是面对成千上万的候选材料。传统的材料开发模式通常为试错法,这种方法耗时良久,且对于一些成因复杂的特性往往难以成功。在上述背景下,机器学习(machine learning)技术被引入到材料设计和材料数据库的构建中来,高效的计算和筛选效率使得机器学习技术成为材料设计的理想“助推器”。机器学习算法的优势在于,其本质上是基于数据统计规律而非物理化学定律的,从而能够绕过复杂的量子力学求解过程。该报告将通过三个例子展示机器学习结合密度泛函理论,在快速发现稳定、无铅光伏钙钛矿、二维铁磁材料、二维固氮电催化剂以及电催化描述符开发等方面的应用。
主讲人简介:
王金兰,东南大学物理学院首席教授、国家杰出青年获得者、国务院政府特殊津贴专家、英国皇家化学会会士。长期从事新材料的多尺度模拟与理论设计工作,在新材料的生长与物性调控、机器学习预测新材料等方面取得了重要进展。在包括Nature、Nature Nanotechnology、Nature Communication等有影响力的期刊上发表论文200余篇,引用一万六千多次,Elsevier中国高被引学者。担任Nanoscale副主编、Nanoscale Horizons科学编辑及Journal of the Physical Chemistry Letters等多个期刊的编委。
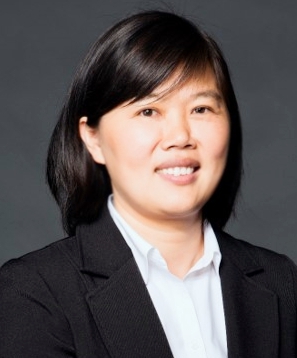
笼目金属材料探索和关联拓扑效应研究
主讲人:雷和畅教授
报告摘要:
笼目(Kagome)晶格因为具有非平庸的拓扑电子结构,比如狄拉克节点、平带和鞍点等,以及阻挫磁性而引起了人们的广泛关注。该报告将简要介绍雷和畅教授团队对于Fe3Sn2, Co3Sn2S2, YMn6Sn6、AV3Sb5(A = Rb and Cs)等几类笼目金属拓扑电子结构和能带拓扑与自旋、轨道等自由度耦合导致的新颖拓扑物态方面的研究结果。这些研究工作表明笼目金属体系已经成为研究关联拓扑效应的一个重要平台,而在其中有更多新奇的拓扑物态有待人们去探索和发现。
主讲人简介:
雷和畅,中国人民大学物理学系教授、博导,国家优秀青年基金获得者。2009年毕业于中国科学院合肥物质科学研究院固体物理研究所,获得凝聚态物理学博士学位。2009-2012年和2012年-2014年期间分别在美国布鲁克海文国家实验室Cedomir Petrovic研究组和日本东京工业大学Hideo Hosono研究组从事博士后研究。2014年底回国,在中国人民大学物理系任职,主要从事实验凝聚态物理研究,包括拓扑量子材料、新型超导材料和低维磁性材料的探索制备、晶体生长和物性研究等。目前,在《Nature》、《Nat. Phys.》、《Nat. Commun.》、《Phys. Rev. Lett.》、《Phys. Rev. X》、《J. Am. Chem. Soc.》等期刊上发表研究论文200余篇,至今这些论文被引用超过5000次。
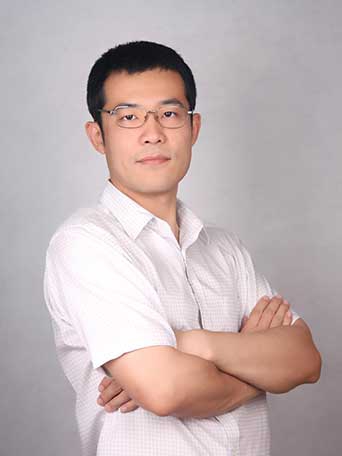
Spin group symmetry in magnetic materials and its application on new topological phases
主讲人:刘奇航副教授
报告摘要:
Symmetry formulated by group theory plays an essential role with respect to the laws of nature, from fundamental particles to condensed matter systems. In this talk, we elucidate that the crystallographic symmetries of a vast number of magnetic materials with light elements, in which the neglect of relativistic spin-orbit coupling (SOC) is an appropriate approximation, are considerably larger than the conventional magnetic groups. Thus, a symmetry description that involves partially-decoupled spin and spatial rotations, dubbed as spin group, is required. We then derive the classifications of spin “point groups” describing coplanar and collinear magnetic structures, and the irreducible co-representations of spin “space groups” illustrating more energy degeneracies that are disallowed by magnetic groups. These results directly give rise to our further discovery of SOC-free topological phases, including Z2 topological classification and topological semimetals, protected by new symmetries.
主讲人简介:
刘奇航,南方科技大学副教授,本科及博士均毕业于北京大学物理学院,曾任美国西北大学博士后、美国科罗拉多大学博尔德分校助理研究员。主要从事计算凝聚态物理研究,研究兴趣包括理解材料中新奇的电学,磁学,光学,缺陷,自旋极化,拓扑等物性,以及功能导向的新型材料设计及预测。发表SCI论文70余篇,H指数28。2018年作为独立PI后发表通讯作者文章26篇,包括6篇PRL、4篇PRX、3篇Nat. Commun.等。2018年获中组部青年千人计划优先支持(理论200万经费),2019年入选广东省珠江人才计划(青年拔尖),2020年作为项目负责人获批国家重点研发计划“量子调控与量子信息”重点专项(青年项目)。2021年入选斯坦福发布的全球前2%顶尖科学家榜单(World’s Top 2% Scientists)。
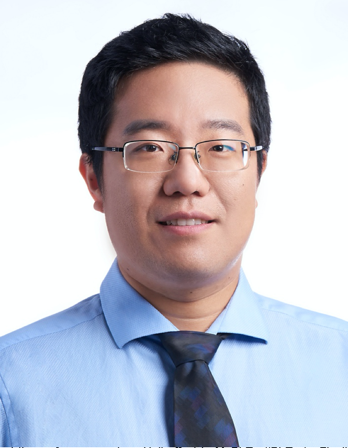